Data annotation has become an essential part of modern artificial intelligence development. As the backbone of machine learning models, annotated data plays a crucial role in improving accuracy, reliability, and overall performance. In this article, we will explore the world of data annotation technologies, providing you with in-depth insights and expert reviews to help you make informed decisions.
Data annotation tech reviews are critical for businesses and individuals seeking to harness the power of AI. This process involves labeling raw data such as images, text, audio, and video to make it machine-readable. By doing so, developers can train algorithms to recognize patterns and make accurate predictions.
This guide will cover everything you need to know about data annotation tools, techniques, and best practices. Whether you're a beginner or an experienced professional, this article aims to provide valuable information to enhance your understanding of data annotation and its role in AI development.
Read also:Caitlin Clarks Impact And Journey In European Basketball
Table of Contents
- Introduction to Data Annotation
- Data Annotation Technologies
- Top Data Annotation Tools
- Annotation Methods and Techniques
- Challenges in Data Annotation
- Data Annotation Tech Reviews
- Applications of Data Annotation
- The Future of Data Annotation
- Best Practices for Data Annotation
- Conclusion
Introduction to Data Annotation
What is Data Annotation?
Data annotation refers to the process of adding meaningful labels to raw data to make it understandable for machine learning algorithms. This process is vital for training supervised learning models, which rely on labeled datasets to learn patterns and make predictions.
The importance of data annotation cannot be overstated, as it directly impacts the performance and accuracy of AI models. High-quality annotated data ensures that algorithms can recognize patterns effectively, leading to better decision-making capabilities.
Data Annotation Technologies
Types of Data Annotation Technologies
There are various technologies used in data annotation, each tailored to specific types of data. Below are some of the most common technologies:
- Image Annotation: Used for labeling images for computer vision tasks such as object detection and facial recognition.
- Text Annotation: Involves labeling text data for natural language processing (NLP) applications like sentiment analysis and named entity recognition.
- Audio Annotation: Focuses on labeling audio files for speech recognition and transcription tasks.
- Video Annotation: Combines image and audio annotation to label video data for applications like autonomous vehicles and surveillance systems.
Top Data Annotation Tools
Popular Data Annotation Tools
Several tools are widely used in the data annotation industry. Here are some of the top tools:
- Labelbox: A versatile platform for image, text, and video annotation with customizable workflows.
- SuperAnnotate: Offers advanced annotation features and integrates seamlessly with machine learning pipelines.
- Amazon SageMaker Ground Truth: Provides automated data labeling capabilities with human review options.
- V7: A powerful tool for 3D and 2D image annotation, ideal for autonomous driving and robotics applications.
Annotation Methods and Techniques
Common Annotation Techniques
Data annotation involves several methods, each suited for different types of data and use cases. Some of the most common techniques include:
- Bounding Boxes: Used for object detection in images by drawing rectangles around objects of interest.
- Segmentation: Involves dividing an image into multiple segments or regions to provide more detailed annotations.
- Transcription: Converts audio or video content into text for natural language processing tasks.
- Named Entity Recognition: Identifies and categorizes key information in text, such as names, locations, and dates.
Challenges in Data Annotation
Overcoming Annotation Challenges
Despite its importance, data annotation comes with several challenges. Some of the most significant challenges include:
Read also:Unveiling The Remarkable Journey Of Matthew Yearick A Visionary In His Field
- Data Quality: Ensuring high-quality annotations is crucial for training accurate models, but it can be time-consuming and expensive.
- Scalability: As datasets grow larger, managing annotation tasks becomes increasingly complex.
- Consistency: Maintaining consistent labeling across large datasets is essential for model performance.
Data Annotation Tech Reviews
Expert Reviews of Data Annotation Technologies
Based on extensive research and user feedback, here are some expert reviews of popular data annotation technologies:
- Labelbox: Highly praised for its user-friendly interface and robust annotation capabilities, making it an excellent choice for both beginners and professionals.
- SuperAnnotate: Offers advanced features and seamless integration with machine learning workflows, ideal for complex annotation tasks.
- Amazon SageMaker Ground Truth: Provides automated labeling capabilities, reducing the need for manual annotation and improving efficiency.
Applications of Data Annotation
Real-World Applications
Data annotation has numerous applications across various industries. Some of the most prominent use cases include:
- Healthcare: Used in medical imaging analysis to detect diseases and improve diagnostic accuracy.
- Autonomous Vehicles: Essential for training self-driving cars to recognize objects and navigate safely.
- Retail: Applied in inventory management and customer behavior analysis to enhance shopping experiences.
The Future of Data Annotation
Trends and Innovations
The field of data annotation is continuously evolving, with new trends and innovations emerging regularly. Some of the key trends include:
- Automation: Increasing use of automated annotation tools to reduce human effort and improve efficiency.
- AI-Assisted Annotation: Leveraging AI to assist human annotators, enhancing accuracy and speed.
- Cloud-Based Solutions: More tools are moving to the cloud, offering greater flexibility and scalability.
Best Practices for Data Annotation
Guidelines for Effective Data Annotation
To ensure successful data annotation projects, consider the following best practices:
- Define Clear Objectives: Clearly outline the goals and requirements of your annotation project.
- Choose the Right Tools: Select tools that align with your specific needs and budget.
- Train Annotators: Provide comprehensive training to ensure consistent and accurate annotations.
Conclusion
Data annotation tech reviews play a critical role in the development of AI models, providing valuable insights into the tools and techniques available. By understanding the importance of data annotation and following best practices, businesses and individuals can harness the full potential of AI.
We encourage you to share your thoughts and experiences in the comments section below. Additionally, feel free to explore other articles on our site for more in-depth information on AI and related technologies.
Data sources and references:
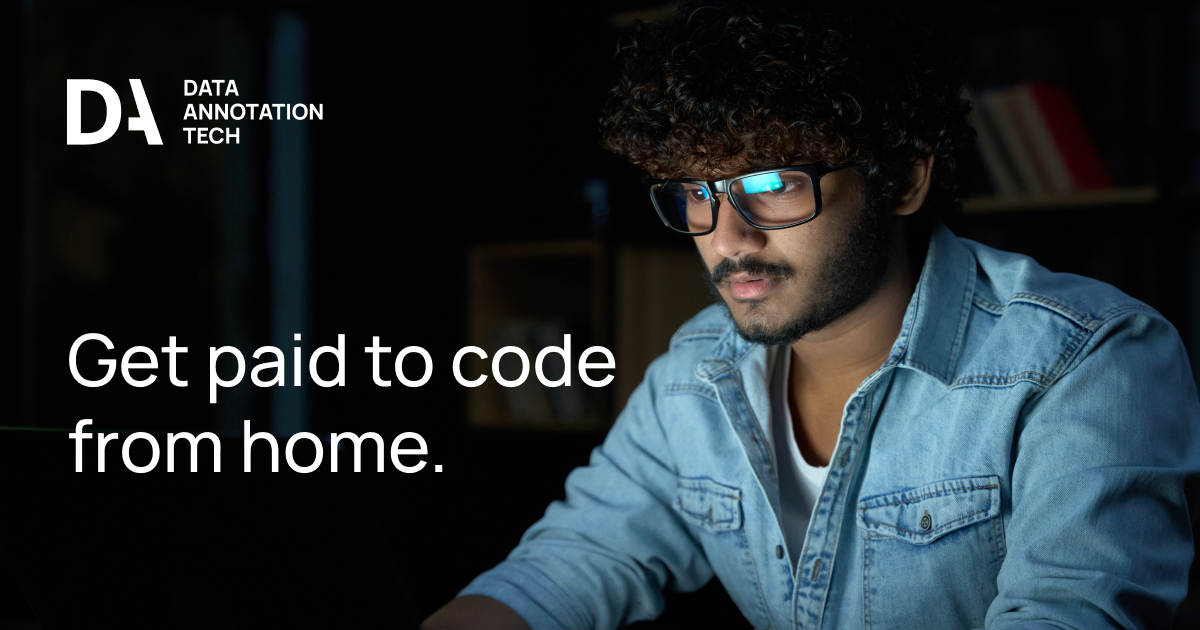
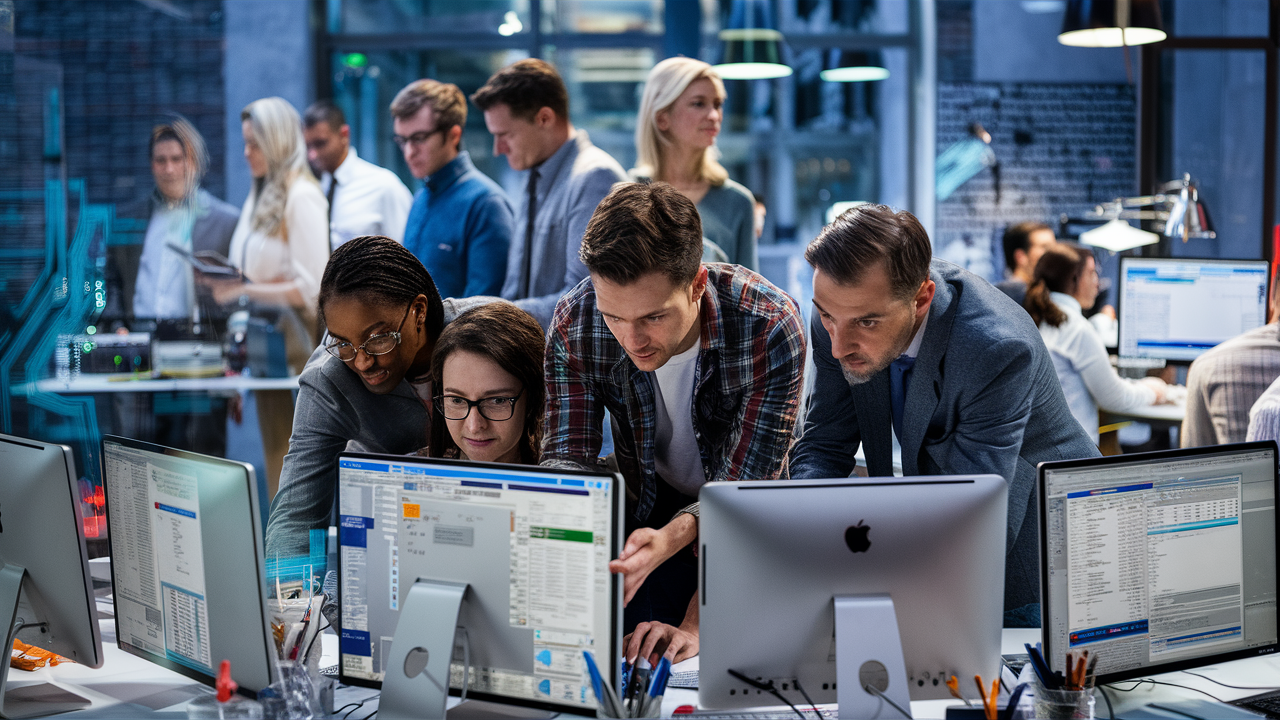
